Pursuing a Data Science Career
If you’ve clicked on this link it’s obvious that you’re someone who’s looking to get into the Data Science career. Like any aspiring data science learner would, you want to know what it’s like after you get into the challenging field of data science. That’s a good thing because you can plan what skills to learn, what kind of Data Science syllabus is right for you, and other pre-requisites.
You might have read about many resources talking about why Data Science is so much in demand, why you should become a Data Scientist, etc. That’s the positive side. But there’s also a negative. This post will shed some light on the negative side focusing specifically on the challenges you may face in the Data Science domain.
Table of Contents
Challenges in Data Science and how the Right Data Science Syllabus will Help
Before we get to what you’ve come looking for, let’s quickly go through some points that are important to set the context right, to know about the challenges in Data Science.
What is Data Science?
Data Science is a field that is responsible to make better use of data. With everything computerized, there’s a lot of data that’s being generated every second. Some of this data is well organized and ready to use. But the rest is in its raw form, meaning there’s not much value for it before it’s extracted and processed. And Data Science is used to make this raw data into readable and valuable data.
Data Science is a combination of tools, technologies, and algorithms working in collaboration to find hidden patterns in raw data. Once found, the data will be extracted, processed, and used to get information that would be helpful for a business or a product.
Value of Data Science in your Resume/CV
There are 2 main ways Data Science can be a part of your resume/CV:
- Data Science Courses/Certifications
- Experience in Data Science
Data Science Courses/Certifications
Having Data Science courses on your resume/CV shows the recruiters your interest in the domain. You wouldn’t complete a course if you weren’t interested. Most of the courses have a great data science syllabus covering theoretical as well as practical aspects. You will have to complete assignments and projects to complete the course. If you’ve completed such high-quality courses from well-known institutes then it adds even more value to your resume/CV. While courses represent your preparation and interest, certifications represent your skills. When you’ve successfully got certified, it shows that you have enough skills to get into Data Science.
Data Science Experience
If you are someone who already works in the Data Science domain, your experience will help you upskill and get better jobs. Mentioning your experience will help the recruiters know what tools, processes you already know, and how ready you are to get a more advanced job.
Adding Data Science experience is only possible for people who are already in the Data Science domain. But courses and certifications apply to everybody: a fresher, experienced person willing to switch into Data Science, or Data Science experienced professional who is looking to advance in their career.
Challenges You would Face in a Data Science Career
Although Data Science is a promising career path and looks like paradise, the ride might get bumpy sometimes. To understand why, let’s look at some of the common challenges you would face in Data Science.
Understanding of the Domain
The definition of Data Science is vague. Due to which there’s a lot of uncertainty when it comes to understanding the tasks involved. This is a challenge especially for freshers who’ve just stepped into Data Science. Freshers are hired based on their eligibility and skills but they’d know the scope of what they should be doing only after spending some time. As you spend more time in the domain, the scope and domain knowledge keeps on getting clear. However, this isn’t a problem for experienced professionals.
Without the proper understanding of the domain, it can get difficult to obtain the desired results. Training can help to a certain extent. But only experience will build a sound knowledge of deciding the right thing to do for the right situation.
Getting the Data that Matters
You can find data in abundance. But what matters is how relevant this data is to you. You can’t find ships sailing at an airport. Similarly, you can’t find the solutions to your problem if you don’t have the right data. And due to data abundance, it can also be difficult to decide whether a dataset would have valuable data that are needed to solve your problem. You can be sure of these only after the initial stages of data cleansing is done.
There might also be cases where you’ve found data that you think is right. But as you keep on working with it, you realize that the dataset has not got enough relevant data to solve your problem. So, all the time and effort you’ve invested go to waste. The only thing you can get out of such a situation is experience.
Data Processing
Data Science is mostly about how you process data. Processing data is a vital yet critical part of Data Science. And no doubt, it becomes a challenge when you have to process what Data Science professionals usually call “messy data”.
Cleaning data, extracting variables into the right form, etc., is difficult especially when the data has anomalies. And if there’s even a slight mistake in processing it, the whole model would give inaccurate or less accurate results. In some cases, you wouldn’t even know that the processing wasn’t right until you get the final output. God forbid, if that happens, you’ll have a hard time fixing it.
Selecting the Right Algorithm
There’s a lot of resources that explain different algorithms and when is the right time to use it. But when it comes to doing it in real and you have to choose one algorithm out of the stack, you can get stuck.
You can’t compromise with results and so it makes it very important that you choose the best algorithm for a particular situation. And there’s no universal algorithm that works for everything. It all depends on the dataset, and what you want to get out of it.
Integrating Tools
Data Science involves using a set of tools to get the job done. Different tools are built for different purposes. And you’ll often have to integrate tools to build the complete pipeline. Finding tools that are easy to integrate is not a big problem because almost every tool is built keeping flexibility and integration in mind. But that’s on papers.
Things are different when it comes to ground reality. Cross-platform support, network configuration, data formats, and a lot of other things come to play. Although you wouldn’t be directly responsible for most of these, it still is a bottleneck in the process. So integrating tools is also one of the challenges you’d face.
Lack of Understanding about the KPI & Role
You wouldn’t find clear KPIs and tasks defined for a role in Data Science in most of the places. For any role, clear KPIs, responsibilities, tasks, and metrics should be defined. This helps individuals as well as the organization. But is most places where there’s a lack of this understanding, Data Science professionals end up doing what they shouldn’t be doing. This eats up their time which they could use to do something that actually matters and makes a difference.
Security
The whole purpose of Data Science is to get valuable data. This data in most cases is also sensitive and confidential. Hence, security is a major concern. Although there will be specialized security teams or members to take care of security, you as a Data Science professional will have to make sure that the tools your use and the pipeline you use are secure.
Another challenge is when there’s security but you don’t have the permissions to use them. There might be cases, where you need to get some sensitive information to complete your model or make it better. But you wouldn’t be allowed to use it because of security issues.
How will the Right Data Science Syllabus Help?
The bitter truth is that there’s no way to completely eliminate the challenges. But what you can do is prepare yourself to face them. Data Science courses are of great help to prep yourself and that’s why the right data science syllabus matters.
How would you know if a data science syllabus is good enough? A good data science syllabus should at the minimum have the latest content of algorithms, processes, approaches, etc., practical demonstration of most-used tools in data science, assignments, industry-grade projects, and most importantly real-life case studies.
If you’re looking for a Data Science program that has an extensive syllabus, you should check out Springboard‘s career program in Data Science. This program is designed to arm you with the skills required for Data Science and help you land a Data Science job.
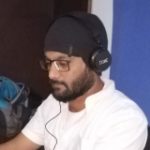
Kamran Sharief
Related posts
Sidebar
Recent Posts
An Inside Look Of Paraulogic
Introduction Welcome to the exciting world of Paraulogic! Are you ready to dive into a linguistic adventure and put your…
Empowering Artists with Cryptocurrency: A Guide to Selling Art Using NFTs
In the ever-evolving landscape of the art world, artists are constantly seeking innovative ways to showcase and monetize their creations….